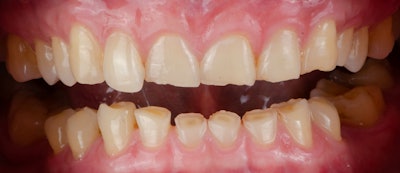
An artificial intelligence (AI)-assisted automated system for detecting tooth wear progression may streamline diagnostics and improve decision-making for tooth restoration. The study was published in the Journal of Dentistry.
Furthermore, the automated method is faster and matches the accuracy of the manual protocol for full-arch intraoral scans (IOSs), the authors wrote.
"The automated measurements of tooth profile loss between IOSs at baseline and at subsequent follow-ups were clinically comparable to measurements using a reliable manual protocol, underlining the potential and validity of fully automated monitoring of tooth wear in a clinical setting, " wrote the authors, led by Niels van Nistelrooij of the Radboud University Medical Center in the Netherlands (J Dent, August 27, 2024, 105323).
The retrospective diagnostic study included eight men with an average age of 43 and generalized tooth wear who were monitored in the Radboud Tooth Wear Project at the medical center. IOSs of the maxillary and mandibular teeth were taken at baseline, with follow-ups at one, three, and five years. The study assessed tooth wear progression by comparing the baseline IOS with follow-up scans, all exported as stereolithography (STL) files.
The automated method segmented the dental arch into individual teeth and used unaffected surfaces for tooth pair registration to track wear over time. It was evaluated against the manual 3D wear analysis (3DWA) protocol by comparing tooth segmentations using the Dice-Sørensen coefficient (DSC) and intersection over union (IoU), they wrote.
The automated method provided a detailed overview of tooth wear progression, with StratifiedTSegNet accurately segmenting each tooth. The largest differences between the automated method and the 3DWA protocol were observed over the zero to five-year interval, where the automated method indicated more tooth wear due to a better fit between corresponding teeth, they wrote.
For example, the automated method showed greater wear on tooth #11's incisal edge, which the 3DWA protocol underestimated due to inaccurate registration. The automated method's segmentation was highly effective (DSC = 0.947±0.033, IoU = 0.907±0.047) and showed a strong correlation with the 3DWA protocol (correlation = 0.932).
Differences between the methods were generally small (95% confidence interval = -0.083 to 0.135), with slightly higher measurements of tooth profile loss for the automated method (mean difference = 0.021 mm, p <0.001). Tooth pair registration failed in 2.9% of cases (15 out of 516) and variability between the two methods increased over longer time intervals, according to the results.
However, the study had limitations. StratifiedTSegNet sometimes failed to segment teeth accurately, possibly due to the algorithm not being specifically designed for worn teeth and the training data being based on colorless plaster models, the authors wrote.
"The proposed automated method for monitoring tooth wear progression was faster and not clinically significantly different in accuracy compared to a manual protocol for full-arch IOSs, " van Nistelrooij and colleagues wrote.