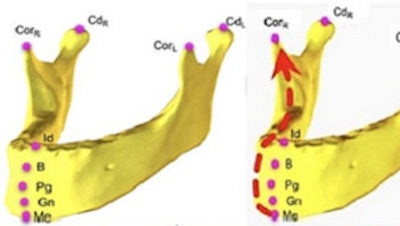
A novel artificial intelligence (AI) technique can accurately locate important points in the skull, face, and jaw bones in computed tomography (CT) scans, suggest findings published on March 6 in the Journal of Medical Imaging.
Researchers, led by Neslisah Torosdagli from Northwestern University in Chicago, found that with their fourfold cross-validation technique, they obtained an average root mean squared error of less than two mm per landmark.
The researchers trained their AI model with a dataset of landmarks derived from an artificially augmented dataset of 250 CT scans from 250 patients. Some of the patients presented with congenital disorders, developmental deformities, missing bones or teeth, and previous surgical interventions.
The team reported "remarkable" accuracy with their technique, highlighting that it was "in line with" or better than that of previously reported techniques (J Med Imaging, Vol. 10:2,024002).
The team used a relational reasoning network (RRN) for their AI model's architecture. With RRN, the model learns about local relations between a given set of craniomaxillofacial landmarks. Then, it learns about global relations between each landmark and the remainder of the landmarks.
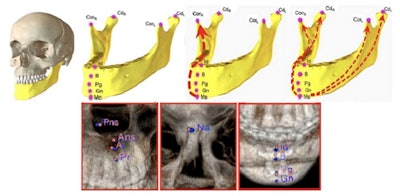
The study authors also highlighted that the model showed good generalizability. They suggested that the results were due to the RRN's ability to learn the functional relationships between craniomaxillofacial landmarks that are still present to some degree in cases of large deformities.
The authors added that accurately identifying anatomical landmarks in this setting is a "crucial step" in analyzing deformities and strategizing for craniomaxillofacial surgeries. They highlighted that more accurate methods, such as their deep-learning technique, could address the limitations of segmentation-based approaches, where segmentation failure could lead to incorrect landmarking.