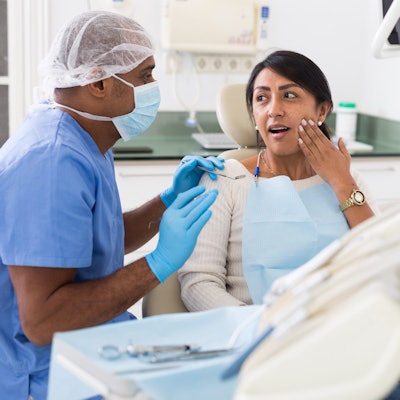
Clinical factors, including pain severity before and during root canals, may better predict the risk of postoperative pain than sociodemographic characteristics such as race and income, according to a study published in Community Dentistry and Oral Epidemiology.
The information may improve the pain management expectations of patients and clinicians, the authors wrote.
"Using clinical factors over sociodemographic characteristics may reduce the sexual/racial biases when predicting risk," wrote the authors, led by Dr. Mi Du of the University of Adelaide in Australia (Community Dent Oral Epidemiol, December 12, 2022).
Improving dental pain prediction risk
Patient characteristics such as sex and age; tooth-level characteristics, including the type of root canal being performed; treatment characteristics, such as instrumentation; and systemic conditions can predict pain following root canals. Though these risk factors are being used to develop numerous models to predict postoperative pain, current research is limited, including that existing literature hasn't compared the prognostic ability of certain characteristics (e.g., clinical and sociodemographic domains).
To better understand which patients are more likely to develop pain after root canal treatment, the study's authors developed predictive models for one-week acute pain and six-month persistent pain following a root canal procedure. Additionally, sociodemographic characteristics, clinical factors, and a combination of both were incorporated into the models for comparison.
The clinical factors used in the models included initial pain quality, how numb patients felt during treatment, the number of days pain kept them from engaging in activities in the past week, and whether pain interfered with their work. Sociodemographic characteristics included the patient's race, their dental insurance status, income, and the sex and ethnicity of the dentist.
Using the U.S. National Dental Practice-Based Research Network, the researchers analyzed data from 707 patients who underwent root canals performed by 62 dental practitioners across six states. Records were collected before treatment, immediately after treatment, one week after tooth obturation, and six months after treatment.
Of the participants, 411 (58%) patients provided one-week post-treatment follow-up pain data, and 651 (92%) provided six-month post-treatment follow-up data. At one week post-treatment, 100 patients (24%) reported a score of 7 or higher for pain after undergoing a root canal. At a six-month follow-up, persistent pain presented in 70 patients (11%), according to the study.
For predicting the one-week post-treatment outcome, a model built with a combined set of predictors yielded the highest area under the receiver operating characteristic curve (AUROC) of 0.85 and area under the precision-recall curve (AUPRC) of 0.72. This was followed by models built with only clinical factors (AUROC = 0.82, AUPRC = 0.66). The lowest predictive ability was found in models with only sociodemographic characteristics (AUROC = 0.68, AUPRC = 0.40), the authors wrote.
In predicting six-month pain outcomes, similar patterns were observed. The AUROC for models with combined, clinical, and sociodemographic sets of predictors was 0.85, 0.89, and 0.66, respectively, and the AUPRC was 0.48, 0.53, and 0.22, respectively, the authors wrote.
The study's authors noted limitations, including that the authors developed and internally validated the models based on an existing cohort and that only patients with access to dental care were included in the study. Before using these models in clinical practices, they should be validated in other populations and tested in clinical settings, they wrote.
Though there is a lack of consensus about how to deal with sociodemographic variables in clinical prediction, the results indicate that clinical factors play a more important role in clinical prediction than sociodemographic characteristics, the authors wrote.
Furthermore, "inclusion of sociodemographic characteristics (e.g., race/ethnicity), clinical prediction may create algorithmic bias, that is enhancing the inequality of healthcare attainment," Du et al concluded.