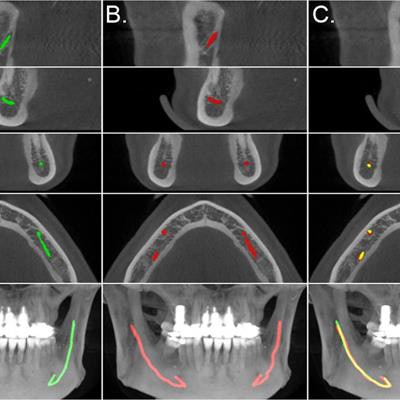
Artificial intelligence (AI) can locate mandibular canals with high levels of accuracy, which may make the dental implant restoration process easier for dentists, according to a recent study published in Scientific Reports.
A deep-learning model that is based on artificial neural networks was able to locate mandibular canals as well as humans can in simple cases that did not involve abnormalities, such as tumors. Automating this process may give clinicians more time to focus on more complex cases, according to the researchers.
"These unparalleled accurate results highlight that deep learning integrated into [the] dental implantology workflow could significantly reduce manual labor in mandibular canal annotations," wrote the group, led by Joel Jaskari, a doctoral candidate at Aalto University School of Science in Finland (Sci Rep, April 3, 2020).
Finding the precise locations of patients' mandibular canals to plan and determine implant size and position before performing procedures is time-consuming. As a result, the researchers began exploring the use of deep learning to automate the process.
Jaskari and colleagues developed their deep-learning method using a dataset consisting of 3D cone-beam CT (CBCT) scans. Using fully convolutional architecture, the model is fast and data-efficient, according to the group.
The study included about 640 dentomaxillofacial CBCT scans from 594 patients. All the scans had isotropic spatial resolution. Radiologists coarsely annotated mandibular canals, using a dataset of 15 volumes with accurate voxel-level mandibular canal annotations for model evaluation.
The prediction accuracy of the deep-learning model was 0.5 mm for about 90% of the mandibular canal length, which is considered more than adequate for dental implant surgery, according to the authors.
The deep neural network model also outperformed statistical shape models, which are the current state-of-the-art method for mandibular canal segmentation. This is an advantage because approaches based on statistical shape models need training annotations to include segmented jawbone, which requires additional manual annotation work or the development of a strong algorithm to segment the jaw, the authors wrote.
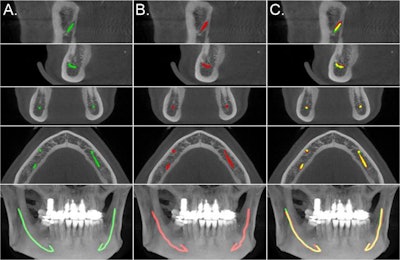
Though no specific limitations were noted, the model's prediction accuracy rapidly dropped when it was used near the mandibular and the mental foramina. However, this was not surprising because these two areas are more difficult to examine due to the anatomy of the human mandible. Nevertheless, the researchers found this decreased performance to be inconsequential as it relates to dental implant restorations because the region near the mandibular foramen is less important and has no teeth.
These results pave the way for other studies to evaluate the model's accuracy using different datasets, such as those including inaccuracies in labeling mandibular canals, and other tasks, such as maxillofacial key-point detection, according to the authors.
"An automated deep learning neural network based system when applied to CBCT scans can produce high quality segmentations of mandibular canals," they concluded.